Recently, Associate Researcher Honglin Liu of the Quantum Optics Laboratory of CAS, in collaboration with Prof. Puxiang Lai's group at the Hong Kong Polytechnic University and Prof. Dawei Zhang from University of Shanghai for Science and Technology, has made significant progress in the mechanism and boundary of applications of imaging through scattering media based on deep learning. The results were published in Photonics Research with the title of “Physical origin and boundary of scalable imaging through scattering media: a deep learning-based exploration”. Convolutional neural network (CNN) is a deep learning method used to reconstruct and classify targets. Since deep learning was first applied to scattering imaging in 2016, it has been a hot spot in the field of optical imaging. In recent years, the structure of neural network has been continuously optimized and upgraded, but imaging through thick scattering media is still out of reach. Moreover, the mainstream of the research direction has been constrained in the development of new networks to improve the reconstruction efficiency, but ignoring the underlying physical foundation.
The researchers used experimental data collected from different areas on the same diffuser, and the simulated data generated by the ballistic light proportional adjustable phasemask (adjustment range 0 % ~100 %) to train and test CNN, respectively. It is found that ballistic light is a prerequisite for the generalization capability of the network to predict through unseen areas, while scattered light function as a specific encoded key, which can be distinguished by the network model under certain scattering condition, i.e., the network can only identify the key seen during training. If there is no ballistic light, even if the data under various scattering conditions are collected for training, the network has no generalization capability to predict through an unseen area.
The discovery of the different roles of ballistic and scattered light in imaging through scattering media explains the physical reason why imaging through scattering media based on deep learning cannot break the bottleneck of thickness. It also provides an important reference and guidance for future applications of imaging through scattering media by deep learning. Increasing the information with spatial invariance can improve the generalization of the neural network, and can also determine the boundary of deep learning-predictive imaging under various scattering conditions.
The project is supported by the National Natural Science Foundation of China, Science and Technology Commission of Guangdong Province.
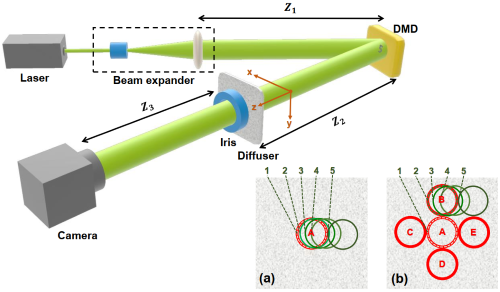
Figure 1 (Image by SIOM)Article website:
https://doi.org/10.1364/PRJ.490125
Contact:
PENG Zexu
General Administrative Office
Shanghai Institute of Optics and Fine Mechanics, CAS
Email: pengzexu@siom.ac.cn
Web: http://english.siom.cas.cn/